Are you struggling to manage an overwhelming flow of data from various sources? There’s a chance you haven’t yet tapped into data ingestion, which could be the game changer you need. This process, a crucial part of the larger data management framework, corrals your dispersed data into a single, cohesive unit.
Such centralization, however, is not just about storage; it's the key to streamlined operations and more informed decision-making. Data ingestion is a transformative option for any organization swamped with data coming from different platforms: it enhances access to this data and offers structured analysis of vast data sets, thereby unlocking new possibilities for your business.
In this article, we’re diving deeper into understanding how data ingestion can be your catalyst for change and growth. If you're ready to transform the way you handle data and gain a competitive edge, read on.
What is data ingestion?
In today's world, where information expands exponentially, data ingestion is becoming a crucial pathway to more efficient and effective data management. At its core, data ingestion is about collecting and funneling raw data from diverse sources into a centralized system. This process is more than just a technical routine; it's the groundwork for turning data into actionable insights.
Imagine data ingestion as a backstage powerhouse, transforming a cacophony of disparate data streams into a harmonious symphony of insights. This transformation is vital in our data-centric era, where information is not just a valuable asset but a cornerstone of innovation and strategic decision-making.
The challenge now is the ever-growing deluge of data. A sophisticated, automated data ingestion process is no longer a luxury but a necessity for organizations. It's the key to centralizing data effectively and maintaining agility in a fiercely competitive landscape. The ultimate goal of data ingestion is to cultivate business intelligence – a beacon guiding an organization's growth and success.
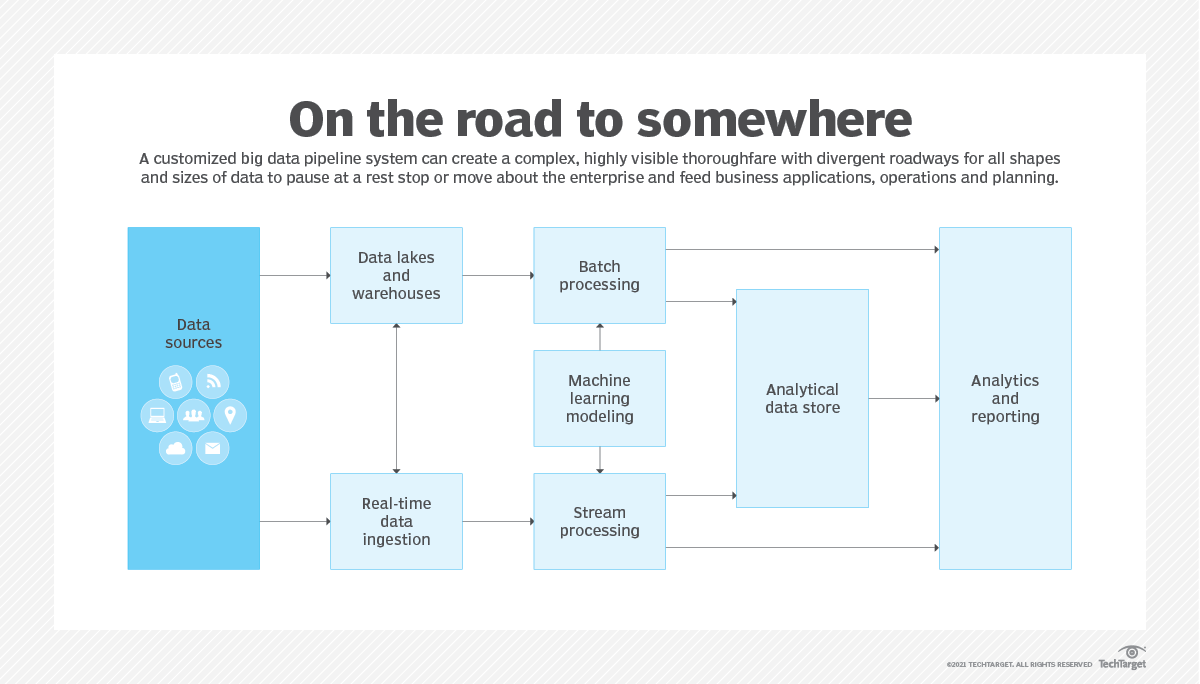
The data ingestion process
Data ingestion is a journey that transforms raw data into a refined, actionable asset. This systematic process plays a critical role in shaping the information your organization relies on through the following:
- Extraction: Imagine this as a mining expedition in the digital landscape. Here, raw data is unearthed from diverse sources such as databases, cloud storage, and external systems. The objective is to uncover valuable information nuggets, preparing them for the next transformative stage.
- Transformation: this stage is akin to an artisan sculpting raw materials. The extracted data undergoes a meticulous process of cleaning, filtering, and enrichment. The goal is to mold the data into a structured, usable format, ensuring its accuracy and alignment with your organization's data strategy.
- Loading (ETL or ELT): With the transformed data at hand, the next step is loading it into a target system, akin to placing a finished sculpture on display. Here, organizations can choose between two primary methods: Extract, Transform, Load (ETL) and Extract, Load, Transform (ELT). ETL involves transforming the data before it reaches its final destination, while ELT involves loading the raw data first and then performing the transformation within the destination system. The choice between ETL and ELT hinges on various factors, including the volume of data, latency requirements, and specific organizational use cases.
By understanding each phase of the data ingestion process, you can better harness the power of your data, turning it into a valuable asset for informed decision-making and strategic planning.
The below video, which explains the streaming applications data ingestion process, offers additional insights into the process:
Types of data ingestion
Data ingestion comes in various forms, each tailored to different needs and scenarios in the realm of data management:
Real-time data ingestion
This approach prioritizes immediacy and agility in data processing. Data is ingested and analyzed instantaneously upon arrival, facilitating rapid decision-making and responsiveness. Real-time data ingestion is essential in scenarios where timely insights are critical, such as in monitoring dynamic social media trends or in overseeing Internet of Things (IoT) device networks. It offers an expedited pathway to actionable intelligence, enabling organizations to respond swiftly to evolving situations.
Batch-based data ingestion
In contrast to the instantaneous nature of real-time ingestion, batch-based data ingestion is characterized by its systematic aggregation and processing of data in predetermined intervals or batches. This methodology excels in managing and processing large volumes of data with efficiency. Although it lacks the immediacy of real-time processing, it is invaluable in contexts where comprehensive, in-depth data analysis is prioritized over instant data accessibility.
Lambda architecture-based data ingestion
This sophisticated approach integrates the immediacy of real-time data ingestion with the thoroughness of batch processing. It utilizes a bifurcated processing pathway, wherein data is ingested concurrently for immediate analytics and stored for subsequent in-depth batch analysis. The Lambda architecture offers a balanced and flexible solution, adept at catering to diverse and complex data processing requirements. It provides organizations with a comprehensive framework that is both scalable and adaptable, crucial for navigating intricate data environments.
Benefits of data ingestion
Data ingestion is a critical step in data management and analytics, offering substantial benefits in terms of efficiency, decision-making, and strategic planning:
Enhanced data quality and consistency
The data ingestion process is instrumental in ensuring that data enters the system in a standardized, cleansed format. This rigorous approach significantly improves data quality and maintains uniformity across the entire dataset. Such consistency is crucial for conducting reliable and accurate analyses, forming a robust foundation for all subsequent data operations.
Streamlined data accessibility and usability
By centralizing and structurally organizing data from various sources, data ingestion markedly increases data accessibility and usability. This streamlined access empowers decision-makers at all organizational levels to derive actionable insights seamlessly. The elimination of data silos cultivates a more informed and collaborative environment within the organization, enhancing overall operational efficiency.
Empowerment of real-time analytics and decision-making
Real-time data ingestion offers unparalleled agility, facilitating immediate analytics and enabling swift decision-making. This capability allows organizations to rapidly adapt to market changes, seize opportunities, and address challenges proactively. It represents a significant shift from retrospective to forward-looking, data-driven decision-making approaches.
Enabling advanced machine learning and AI applications
At the heart of machine learning and artificial intelligence applications lies data. Data ingestion plays a critical role in supplying these technologies with structured, up-to-date data streams. This steady supply of quality data is essential for effectively powering ML and AI algorithms, unlocking new horizons in technological advancement and application for organizations.
Solidifying data governance and compliance frameworks
Data ingestion also lays the groundwork for robust data governance and compliance. By standardizing data processing and ensuring high data quality, organizations can more confidently adhere to regulatory standards. This aspect of data ingestion not only mitigates the risks associated with non-compliance but also reinforces trust in the organization's data accuracy and security protocols, which are paramount in today's data-centric world.
7 data ingestion challenges
Here are the primary concerns pertinent to data ingestion, as well as potential solutions:
1. Ensuring data quality and consistency
A major hurdle in data ingestion is maintaining uniformity and accuracy across different data sources. Discrepancies can arise due to inconsistent formats and incomplete cleansing, necessitating robust validation and data profiling tools for anomaly detection and correction.
2. Scalability and performance management
With escalating data volumes, scalability is imperative. Traditional methods may falter under the pressure of increased data flow, leading to performance issues. Embracing scalable architectures and distributed processing is vital to manage this influx efficiently.
3. Complexities of real-time processing
Implementing real-time data ingestion is complex due to its requirements for low latency and high throughput. This necessitates advanced technology solutions and meticulous architectural design to fully benefit from instant analytics.
4. Data security and privacy concerns
Protecting data security and privacy during ingestion is critical. The process heightens the risk of breaches and unauthorized access, making robust encryption, strict access controls, and adherence to data protection regulations fundamental.
5. Integrating diverse data sources
The variety of data sources, each with its own format and structure, presents integration challenges. Developing connectors and protocols that can handle diverse data formats is essential for a seamless ingestion process.
6. Latency issues in batch processing
Batch processing, while effective for large data volumes, introduces latency. For organizations needing timely insights, balancing the efficiency of batch processing with the need for lower latency is crucial.
7. Monitoring and error handling
Effective monitoring of the data ingestion pipeline is essential to quickly identify and rectify issues. Implementing robust monitoring tools and error-handling mechanisms is key to ensuring the reliability and integrity of the ingestion process.
Data ingestion in action: use cases
Data ingestion plays a crucial role in various real-world scenarios, enabling organizations to leverage their data assets for strategic decision-making, operational efficiency, and innovation. Here are key use cases:
- E-Commerce transaction monitoring: e-commerce platforms utilize data ingestion for tracking purchase behaviors and detecting fraud. This allows for rapid analysis and decision-making, crucial for protecting both customers and business interests.
- Social media analytics: through real-time data ingestion, organizations can monitor social media trends, assess brand sentiment, and dynamically engage with audiences. This real-time analysis is instrumental in shaping responsive marketing strategies.
- IoT sensor data management: in sectors like manufacturing and logistics, data ingestion facilitates the collection, processing, and analysis of sensor data. This capability is essential for operational optimization, predicting equipment failures, and boosting overall efficiency.
- Healthcare patient monitoring: the healthcare industry employs real-time data ingestion in wearable devices and medical sensors, providing continuous data streams for immediate patient health insights. This approach aids in early anomaly detection and the development of personalized treatment plans.
- Financial fraud detection: financial institutions leverage real-time data ingestion to identify unusual transaction patterns and potential fraud, enabling rapid response measures to protect their ecosystem and clients.
- Predictive maintenance in manufacturing: real-time ingestion of equipment sensor data allows for predictive maintenance, enabling proactive scheduling and minimizing downtime. This approach enhances operational efficiency and reduces maintenance costs.
Supply Chain optimization: data ingestion offers comprehensive visibility into supply chains by analyzing data from various sources. This insight is crucial for real-time inventory management, demand forecasting, and optimizing logistics, leading to improved supply chain resilience and efficiency. Learn more about the role of business intelligence for supply chain.
Best data ingestion tools
Data ingestion tools are integral in transferring information from diverse sources into a centralized system, typically a data warehouse. These tools, particularly those with ETL (Extract, Transform, Load) capabilities, are pivotal for efficient data processing. The most advanced tools in this domain not only automate tasks but also offer pre-built integrations and, in some instances, reverse ETL functions. Consider these leading solutions:
VMware Aria Operations for Applications
Formerly known as Tanzu Observability by Wavefront, Aria Operations is renowned for its high-performance streaming analytics for data ingestion, storage, visualization, and monitoring. Its scalability is a standout feature, processing millions of data points per second. Key functionalities include advanced charting, custom alerts, and the Wavefront Query Language (WQL). Pricing is tied to VMware plans.
Apache Kafka
This open-source stream-processing software is lauded for its sophisticated ETL capabilities. It enables the creation of real-time data pipelines from varied sources, boasting high throughput, scalability, durable storage, and a rich open-source ecosystem. Apache Kafka is a versatile choice for automated data ingestion.
Matillion
A cloud-based data integration and transformation platform, Matillion is noted for its flexibility and rapid deployment. It offers features like automation of repetitive tasks, enhanced security, and a comprehensive library of pre-built connectors. Matillion's pricing model ranges from a free tier to usage-based plans.
Amazon Kinesis
This fully managed, cloud-based service excels in real-time processing of large-scale streaming data. It comprises components for video streams, data streams, firehose, and analytics, and integrates seamlessly with other AWS services. Amazon Kinesis is designed to handle terabytes of data per hour and is scalable to suit various needs, with pricing dependent on usage.
Airbyte
As an open-source data integration platform, Airbyte specializes in ELT pipelines. It offers log-based incremental replication and more than 300 ready-to-use connectors, along with support for custom connector development. Airbyte is free to use, with a paid option starting at $2.50 per credit, offering additional features and cloud hosting.
The future of data ingestion
The future of data ingestion is profoundly influenced by technological advancements, with cloud-based tools becoming increasingly central due to their scalability, flexibility, and accessibility. As a result, more organizations are adopting cloud-native data ingestion software, utilizing platforms like AWS, Azure, and Google Cloud to elevate their information management systems. This shift is complemented by the emerging data mesh architecture, which decentralizes data management, allowing individual teams to autonomously manage their specific data domains.
The growing need for immediate insights is pushing data ingestion towards swifter, more dynamic solutions. Technologies like Apache Kafka and Amazon Kinesis are spearheading the move towards real-time streaming, a development that is particularly vital in sectors that require rapid responses, such as finance, healthcare, and the Internet of Things (IoT). In parallel, the incorporation of edge computing into data ingestion is accelerating. By processing data closer to its origin, edge computing not only minimizes latency but also boosts efficiency and supports applications that depend on nearly instantaneous decision-making.
However, the evolution of data ingestion extends beyond just technological developments. An equally important aspect is data democratization, which focuses on making data accessible to a broader range of users, including those with limited technical expertise. Tools like Matillion and Airbyte are enabling self-service data access, allowing users across an organization to leverage data insights independently. This movement towards democratization ensures that data-driven insights are not exclusively available to specialized data teams but are disseminated throughout the organization. This promotes a culture where decision-making is deeply rooted in data, fostering an environment where all stakeholders are empowered by accessible, actionable information.
Need help implementing data ingestion best practices?
As we conclude this exploration of data ingestion, it becomes clear that the process isn't just something to consider, but rather a strategic play in the data-driven environment. If you decide to make data ingestion a part of your organization's operations, our team at RST Software is ready to assist with best practices. With a wealth of expertise and a commitment to transforming data into a strategic asset, we are well-positioned to tailor solutions that align with your unique needs. Contact us today and let’s turn data from a resource into a catalyst for insights and growth.